Few topics have captured the minds of technologists like Machine Learning (ML) has over the past few years. Walk through the booths at CES and you will find many new products and services promising to utilize ML in some innovative way to help distinguish their offering from the next.
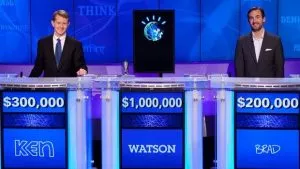
IBM's Watson used machine learning to defeat two Jeopardy contestants.[/caption] That has certainly been the case for cybersecurity platforms. As malware has expanded outside the domain of script kiddies, it is increasingly being used to turn a profit by more organized criminal organizations. Traditionally, the professionals have utilized antivirus signatures to identify and extricate the most common forms of malware. But the complexity and proliferation of malware make it more difficult to detect. The most complex malware today can evade signature detection. This is where heuristics can help. And one of the most promising forms of heuristic technology is machine learning malware analysis. There is a lot of confusion about the role of machine learning and artificial intelligence will play in combating malware. Some people use machine learning and artificial intelligence terms interchangeably, but they should not. Both ML and AI play crucial roles in fighting malware, and it is important to understand how they differ.
The Differences between Machine Learning and Artificial Intelligence:
- Machine learning is an algorithm that recognizes patterns in large amount of data.
- Machine learning focuses on completing pre-programmed tasks.
- Artificial intelligence is when a computer learns to “think” for itself.
- A computer learning to play chess by analyzing previous games it has played is an example of artificial intelligence.
Behavior Modeling
Malware detection tools have traditionally relied on trapping or bad-behavior models. Security tools trapped threats by matching malware signatures to databases of known harmful code. Trapping malware in this fashion worked well before the threats become more sophisticated and avoided signature detection. Malware authors figured out they could write single-use malware that had never been seen by the security community to inflict substantial damage. Machine learning allows us to move from trapping to hunting malware based on behavior modeling. Bad-behavior modeling looks at actions such as accessing saved passwords, local documents, browsing history, or contacts. Malware detection tools were designed to act only when they detected this type of behavior. The tools could only act on what they were programmed to do. They could not “learn” on the fly. Hunting models use good-behavior modeling that is much more difficult to circumvent. Good-behavior modeling uses machine learning to determine when an employee is most likely to login to the network or access certain file shares. It may also detect the following:
- An employee or device transferring copious amounts of data.
- Making a connection to another network or device that is outside normal use or normal hours.
- Using programs and tools that are outside the employee’s domain. For example, an employee from accounting running a network scan tool during the evening.
- An employee or device that is using an excessive amount of computer resources such as CPU, GPU, or memory.
Good-Behavior Modeling Relies on Machine Learning
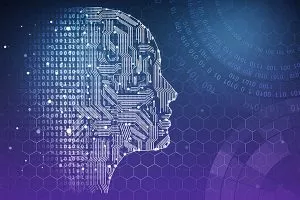
Developing tools based on good-behavior modeling is a big task because it requires the capturing, analysis, and processing of massive amounts of data. Computers then classify everything that users and programs should do. When we know what actions are considered “good”, we know everything else must be “bad”. We not only have to have access to the data, but we also need computers with enough processing power to perform the necessary fabrication. Cloud-based services has made this processing power more affordable which encourages more companies to join in the fight. Another challenge is that data processing never stops. Because human behavior changes, the behavior-modeling must change, or it becomes obsolete. This allows the detection tools to “learn” on the fly and increase in intelligence as it processes more data.
Conclusion
Malware is becoming more advanced and more nefarious. Tools we have relied on in the past will not work in today’s computing environment. While machine learning and artificial intelligence will certainly play a key role in combating malware, it will also be used by malware authors to make their attacks more difficult to detect. It will take the collective efforts of the cybersecurity community to stay ahead of these sophisticated threats. As you consider products to keep your networks safe, look for those that utilize machine learning and artificial intelligence to help stay ahead of the curve.
You May Also Like
- Compliance Cybersecurity Data Protection Data Resilience Ransomware
Cybercriminals Are Already Leveraging AI to Get to Your Data. What Can You Do About It Today?
June 13th, 2024 - Backup and Disaster Recovery Business Continuity Compliance Cybersecurity Data Protection Data Resilience Ransomware
Unified Backup and Disaster Recovery Solutions: The Crucial Balance Between Data Security, Accessibility, and Compliance
May 9th, 2024 - Business Continuity Cybersecurity Data Protection Data Resilience Ransomware
What Is Quantum Encryption, How Does It Work, and Will It Save Us From Cybercriminals?
April 30th, 2024